“Good health and well-being” is one of the 17 UN Sustainable Development Goals (SDGs) and is a fundamental human right. According to a study conducted by The Lancet, while India’s ranking on the global Healthcare Access and Quality (HAQ) Index improved from 153 in 1990 to 145 in 2016, the country’s score of 41.2 points is below the global average and worse than some of the poorest regions in the world. On a more regional scale, India ranks 10th out of 11 Asia-Pacific countries in the new Personalised Health Index.
India’s National Health Policy (2017) aspires to attain the “ highest possible level of health and well-being for all and for all ages through a preventive and promotive health care orientation in all developmental policies, and universal access to good quality health care services without financial hardship to the citizens.” However, the COVID-19 pandemic shed light upon several fractures underlying our healthcare system- some unforeseen and some previously ignored. During the first lockdown, India, along with the rest of the world, was ill-prepared to combat a national health emergency, and its swift but draconian measures led to adverse economic effects that have been vastly discussed and debated by policymakers and civil society. This called for a heavier investment in the health infrastructure.
In the Union Budget 2021, the government allocated Rs. 2,23,846 crore for health and well-being, an increase of 137% from the original 2020 budget estimate. Of this, Rs. 35,000 crore has been earmarked for the COVID-19 vaccine for 2021-22. Interestingly, an allocation similar to the 14,217 crore provision in 2020-21 for the COVID-19 Emergency Response and Health System Preparedness Package was missing from this year’s budget despite the pandemic. Moreover, all these designations are primarily only for immediate emergency response and are not part of the integral health budget for sustainable long-term impact.
Despite several warnings from experts, the Indian government was severely underprepared for the Second Wave, which resulted in high mortality rates and a breakdown of healthcare infrastructure across the country. There is wide consensus that the number of active cases and deaths have been vastly underreported, with the real toll being significantly higher. A lack of comprehensive guidelines and a health system that was overwhelmed within a few weeks saw citizens scrambling to access medical supplies through any means possible.
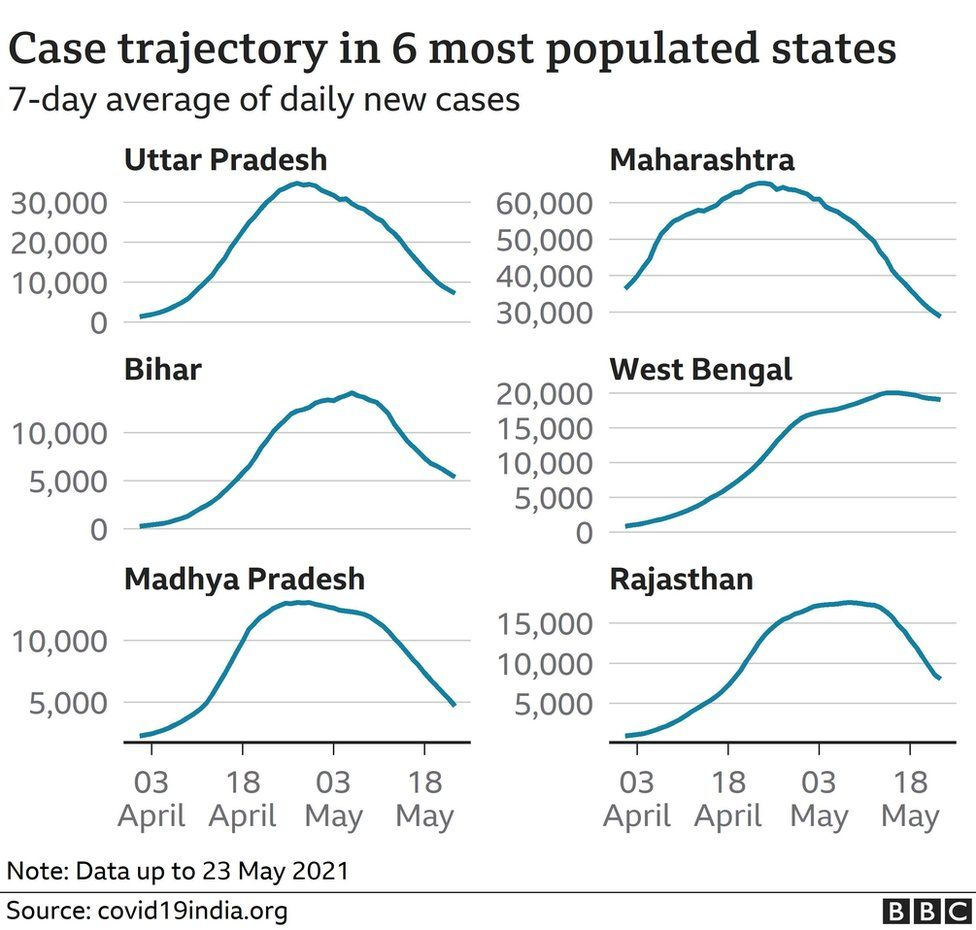
The Second Wave highlights myriad shortcomings on the government’s part- while the funding to construct resilient healthcare infrastructure to prepare for future outbreaks was cleared in April 2020, the tender for oxygen plants was not released until October, and a mere 33 plants were instituted by April 2021. According to ICMR estimates, there were around 70,000 ICU beds, and an even more microscale number of ventilators available. Moreover, the rhetoric espoused by India's self-reliance led to slow approval and purchase of foreign vaccines, while simultaneously exporting doses without making sure that it had enough for its own people.
The availability and development of public health infrastructure is uneven across Indian states. A few states like Maharashtra, Gujarat, and the capital city of Delhi, which are some of the country’s most economically developed regions, faced the brunt of the Second wave. Meanwhile, states like Tripura which are comparatively much less economically competitive showed larger resilience. While there may be multiple explanations behind this phenomenon, the former’s health infrastructure deficiencies can possibly be explained by the fact that their economic growth was not properly accompanied by an even improvement of the quality of life.
Data from the Economic Survey (2020-21) tells us that India ranks 179 out of 189 countries considering healthcare prioritisation in the government budget. India devotes about 1.25% of its GDP on health, and the private sector pays for more than 71% of healthcare in both urban and rural parts. India has almost twice as many private hospitals as public one (an estimated 43,487 vs 25,778) with the private healthcare sector accounting for around 60% of in-patient care.
However, public health services are fashioned based on the required minimum capacity, and thus, the public-private divide is tragically apparent in the wake of the pandemic. For instance, a majority of available ventilators are only in private hospitals and are concentrated in just seven states. The care at private health facilities comes at a high price. There have been reports of private hospitals overcharging desperate patients, and of hospitals billing patients for upto three to four times higher than the prescribed rate. This situation is extremely dangerous considering about 85.9% of India’s rural population and 80.9% of its urban population don’t have health insurance, causing a steep decline in their economic well-being. The prevalence of under-testing and under-reporting means that the gravity of the situation is misrepresented, paving the way for lesser urgency to reform the country’s healthcare infrastructure.
The Second Wave saw the rural economy being crippled, exacerbating urban-rural inequality. It is vital that the government works to strengthen the public healthcare system from the grassroots by investing more in village health infrastructure. On 13th August, the Union Health Ministry released the second part of the ‘India Covid-19 Emergency Response and Health System Preparedness Package’ (ECRP-II) for this very endeavour. The ECRP-II package was launched on July 22nd with a total budget of Rs 23,000 crores to bolster district and rural level healthcare across the nation.
Under ECRP-II, states have been given more than Rs 7000 crores to ramp up their preparedness for the anticipated Third Wave. This includes a blueprint to support the existing number of ambulances with additional ALS and BLS ambulances. District administrations have been tasked with securing a stock of 10,000 kilos of oxygen 24/7, and to have 2.44 lakh beds nationwide for Covid-19 patients (with a minimum of 20% for the ICU). The number of ventilators in the public health system has increased from 17,000 to almost 60,000. Tele-consultation services are being reinforced in 733 district hubs to aid 15,632 people. The scheme aims to increase testing, and public health staff at the grassroots level are to be trained rigorously to use medical equipment in an efficient manner.
While India has fully vaccinated about 11.7% and partially vaccinated 38.4% of the population, it is necessary to speed up and decentralise the vaccination drive, and find ways to bridge the digital divide by making vaccination accessible to everyone eligible visiting their nearest public healthcare centre (PHC). The Tamil Nadu Chief Minister M.K Stalin brough COVID-19 treatment in private hospitals under the purview of a government insurance scheme. It is essential to devise such measures to stop healthcare costs from being prohibitive.
It is imperative for the Indian government to have a long-term vision to deal with the repercussions of a devastating pandemic that shows no sign of disappearing at the present. It is necessary to facilitate the creation of a collaborative environment for public and private healthcare providers to overcome the shortcomings in both sectors. Despite the calamitous Second Wave, people have continued to flout quarantine rules. Hence, a significant part of the onus lies on the healthcare infrastructure to provide curative measures while simultaneously working on prevention via universal vaccination.